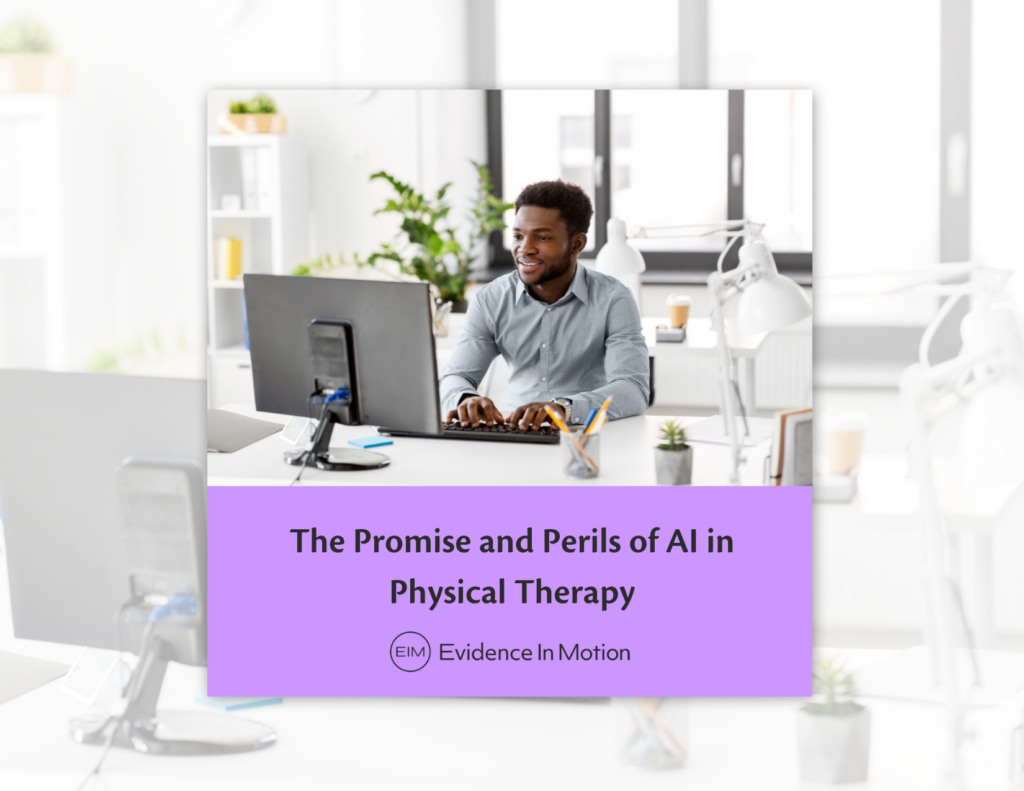
AI is having a moment. Seemingly overnight, AI has shifted from a far-off technology of the future to everywhere all at once. Every company seems to be adding it to their business, every website is posting about it—heck, AI was even the villain in the latest Mission: Impossible movie. And based on my own experience, it has also caught the attention of PT students.
During a recent talk with a group of students from the University of Kansas DPT program over Zoom, I made a brief mention of ChatGPT during a broader conversation about emerging technologies—and then watched as an otherwise subdued chat exploded with comments on and questions about ChatGPT. Students were dying to know how that particular form of AI could be leveraged in PT moving forward and were eager to share their thoughts on its potential applications.
The excitement is certainly understandable; as a profession that continues to suffer from administrative harm, ChatGPT and AI as a whole have the potential to ease portions of the documentation burden and improve the uniformity of care based on objective, research-based clinical pathways. But before diving headlong into this bold new future, I’d like to address several crucial questions to ensure clinicians can effectively integrate AI into their practice.
What is AI?
Let’s start with the most fundamental question: what is artificial intelligence (AI), exactly? The simplest explanation is that AI is a machine that is able to perform cognitive functions that we associate with the human mind such as perception, learning, reasoning, problem-solving, and environmental interaction at speeds that surpass human capabilities. If you are using voice assistants Siri or Alexa, then you are familiar with AI capabilities already. Under that umbrella, there are different types and applications of AI:
- Narrow AI (also called weak AI): As IBM lays out in this primer, narrow AI is built and trained for a simple task or series of tasks. Siri, Alexa, and the bots we at WebPT and many other technology companies employ on websites to analyze data are good examples of narrow AI.
- Strong AI: Comparatively, strong AI is a still-theoretical AI with intelligence truly equivalent to and indistinguishable from what humans possess, with the consciousness to match. In other words, the type of AI that skeptics and doomsayers are really worried about— think Ex Machina, The Matrix, or WALL-E.
There are also branches of AI that you may see referenced in regard to current or emerging technologies that are also worth knowing. Machine learning is the application of AI to mimic human learning and is an important part of AI applications that make use of predictions or data-driven insights. Along those lines, neural networks are the nodes used in machine learning that imitate the neurons in the human brain — hence the “neural.”
Generative AI versus Predictive AI
Now that we’ve defined AI more broadly, let’s look at the differences between the AI we’re already seeing in broad use today. All the available products and solutions fall into two categories: generative AI and predictive AI.
Generative AI uses algorithms to create original content based on the structure and pattern of other similar content that it has studied in response to a prompt. ChatGPT is the most well-known example of generative AI, essentially synthesizing an internet’s worth of examples to create “original” text, images, or video in response to a request made by the user. DALL-E is another example of generative AI that is taking the internet by storm by creating unique art and images based on users’ descriptions.
Predictive AI, on the other hand, looks at a set of data and analyzes it to understand patterns and trends in order to provide informed predictions. Predictive AI is widely used in the world of finance, banking, and healthcare, but an example much closer to home is Netflix, which uses predictive AI to analyze a user’s viewing history and habits—along with those of its hundreds of millions of other users—to suggest what you should watch next. Granted, these concepts are not new in the sense that companies have been using data to automate and influence product workflows for years. So how do both generative and predictive AI have both application and relevance to the livelihood of every PT?
How can rehab therapists use AI in its current forms?
Admittedly, contemplating the incorporation of AI in a rehab therapy practice can evoke fear, amazement, uncertainty, and excitement, especially since our profession is deeply rooted in factual, physical hands-on treatments with our patients. But as you zoom out a bit and look beyond direct patient care, it’s not hard to see glaring areas where AI could be hugely beneficial in helping clinicians and staff. Here are a few examples of AI in practice that therapists should keep in mind.
It can assist with clinical decision-making.
One of AI’s strengths is its ability to analyze a lot of data and pull out patterns suggesting a possible conclusion in a fraction of the time it would take a clinician. While AI can’t take into account intangibles that do not show up as data points, there’s strong evidence that it can provide helpful predictions to help clinicians make more informed decisions.
One study on using machine learning (ML) and neural networks (NN) to aid in clinical decision-making demonstrated 95% accuracy in predicting potential outcomes for neutropenic patients with fever. The study concludes that “The availability of a large amount of EHR data; the use of ML or NN; and the high level of performance of new computers reveal the immense power that AI can wield in shaping the medical landscape for the better. Our team has already witnessed how AI algorithms can make real-time predictions to positively guide clinicians in their decisions towards patient treatment and care.”
According to this post from the Government Accountability Office, human diagnostic errors can affect over 12 million Americans and cost over $100 billion—numbers that machine learning workflows could significantly reduce. Similarly, this study outlines how AI and machine learning can help providers make data-driven decisions to optimize outcomes for objective functions—something that’s sorely needed in clinical care.
Looking at it from a rehab therapy perspective, AI could quickly review a patient’s subjective information to automatically highlight red flags for you to be aware of—say, high blood pressure, allergies, or medication risks. Yellow flags such as diagnosis, chronic versus acute injury date, or insurance type could be used as risk adjustments for scheduling frequency and duration or reminder call prompts for example.
It’s ideal for billing and coding work.
As I mentioned in my previous post, AI appears to be the perfect fit for assisting rehab therapists in coding treatment—a time-consuming but crucial task that ensures proper payment. AI can quickly analyze the diagnosis and treatment provided in a patient’s documentation—a key point — to produce the best codes to bill. Of course, AI isn’t going to get it right every time—which is where machine learning can come in to recognize patterns and suggest the most accurate billing codes. If AI can significantly reduce the tedious work around coding and relieve some of the administrative burden that we are currently facing, it could help us address burnout and improve retention amid the staffing shortage for revenue cycle personnel and clinicians.
It makes marketing and SEO optimization easy.
Unfortunately, rehab therapists don’t get enough of the marketing education we need to run a business—so it makes sense to look to AI for help. With AI-assisted marketing, clinic owners and directors can:
- Analyze the topics most patients are searching for and generate outlines for compelling patient-focused content around those keywords;
- Generate automated email campaigns to engage patients on topics they care about;
- Deploy chatbots on their site to answer questions and direct visitors to the right links; and
- Optimize your site for search.
What should providers be wary of with AI?
Anyone who’s watched enough sci-fi knows that the technology of the future always comes with a catch. Fortunately, using AI to help with medical coding or crafting an email isn’t enough to stoke fears of Skynet, but there are a few flaws in current AI that you’ll need to look out for if you’re considering adding AI to your current clinic workflows.
AI is new—which means it’s still learning.
Since AI is a relatively new technology, it will take time to get it right. AI requires a massive amount of data to “learn,” so to speak—and finding quality data for AI remains a challenge for many AI companies. Without the necessary volume of high-quality data, AI performance suffers.
What makes it even more challenging is that, as this article from BroutonLab points out, AI won’t put its hand up and admit it is uncertain or doesn’t know something, as a human would. Instead, it will complete its operations and provide a less-than-reliable result—and end users would be none the wiser.
Fortunately, clinicians have their own education and expertise to fall back on. To that end, using AI in these early stages requires diligence in checking every answer or suggestion and applying your own critical thinking. (More on that later.) Overconfidence in the reliability of AI at this early stage of its development could be very detrimental to our clinical reasoning and judgment capabilities. That’s why I personally think about AI as a potential co-pilot in clinical practice. It’s there to help me steer the plane, provide valuable insights to help me make more precise decisions, synthesize the historical data in combination with current findings and help make the flight enjoyable and efficient for the passengers—but I am still the captain.
AI is learning from existing data—including its biases.
Speaking of repeating mistakes, another issue AI is facing in its early days is that it’s perhaps learning from humans too well. There’s a wide swath of evidence that implicit bias in health care is creating and exacerbating disparities, and without human intervention, there’s every reason to believe that AI would simply continue these trends. In fact, there’s already one studydocumenting how an algorithm employed by a hospital required Black patients to be much sicker than white patients in order to be recommended for the same treatment—and that predates the AI craze. Conversely, it might ignore important racial or ethnic distinctions between patients that are essential for providers to know to make the most well-informed care decisions.
AI presents a host of HIPAA questions.
For healthcare organizations to train AI to provide better information and predictions, many must provide that AI with access to existing patient records—which opens a Pandora’s Box of privacy concerns. To avoid potential HIPAA violations, every healthcare organization should be deidentifying sensitive information before contemplating the use of any AI platform. As this article in the AMA Journal of Ethics lays out, there’s a risk that the de-identified data could be reidentified due to the analytical power when paired with other data streams, as might happen with AI pulling data from multiple sources.
There’s also an important distinction between using open-source data versus a closed source for data (which is to say our own clinic information). In both instances, if the results are to be published, used in an open forum, or for research those uses should be made clear to patients. However, for efficiency tools or data analytics used internally for your clinic only, there may be fewer data restrictions.
AI might get stuck in a feedback loop.
Currently, generative AI is pulling from mostly human-generated content and data open-sourced on the internet. But AI-generated content is being developed and published at an extremely high rate. What happens when there’s more AI-generated content on the internet than human-generated content? According to one recent study, the possible answer is that AI starts learning from other AI, forgets the original human data over time, and eventually collapses in on itself as it distorts the information and skews data toward the mean rather than fully representing less popular data. In short, AI output risks becoming, as noted in this article, a copy of a copy of a copy—not ideal if you’re relying upon generative AI to help you create unique patient evaluations, for example.
PTs could become complacent.
It’s a spicy take, but hear me out: if rehab therapists get a little too used to having all the answers right at their fingertips, we run the risk of allowing our clinical knowledge to get rusty as those muscles don’t get used as often. That’s not just speculative, either; one study from 2020 shows humans get complacent when working alongside an AI.
On the other hand, recent history suggests that we may get complacent about some things that have been replaced by technology, it opens up the opportunity to explore and store knowledge about other things. After all, how many phone numbers do you actually know by heart these days? So provided that clinicians are handing off rote tasks to AI while maintaining their expertise in areas that machines can’t replicate, it may not prove as much of a concern
AI might be faster than its human counterpart in some areas of pattern recognition or data analysis, but during these early stages of development, it may not always be right. So if providers rely too heavily on AI-generated output without providing oversight and using their clinical judgment, there’s a tremendous risk of diminishing our quality of care. AI-generated predictions or recommendations still require human input to consider comorbidities and other mitigating factors to arrive at the correct plan of care.
While there are certainly some challenges to the early adoption of AI, the genie is out of the bottle, and it’s not going back in. It will be a tool to be utilized to enhance our clinical decision-making, improve the standardization of care and relieve the administrative burden of clinicians and clinic staff. Getting there, however, will require rehab therapists to overcome their fears of being replaced or at least marginalized. David Elton, VP of Musculoskeletal Research and Development at UnitedHealth Group, captured that sentiment when he remarked on the State of Rehab Therapy report that “While there is some potential to improve administrative inefficiencies or improve therapists’ clinical knowledge and skill with AI and VR, respectively, there is the risk of losing the deeply personal, hands-on care highly valued by patients.”
I understand the concern that many clinicians feel about AI, but I think we should be confident that AI can never replicate what we do as clinicians but perhaps enhance our ability to treat more patients and improve the efficiency of our practices. That’s why I think AI is best-suited as our co-pilot in treatment—and like any new addition to the team, there will be a learning curve that requires some extra vigilance on the part of seasoned clinicians.